IEEE Transactions on Neural Networks and Learning Systems
Zhongwei Huang1 Haijun Lei1 Guoliang Chen1 Alejandro F Frangi2 Yanwu Xu3 Ahmed Elazab1 Jing Qin4 Baiying Lei1∗
1Shenzhen University 2University of Leeds 3Chinese Academy of Sciences (Ningbo) 4Hong Kong Polytechnic University
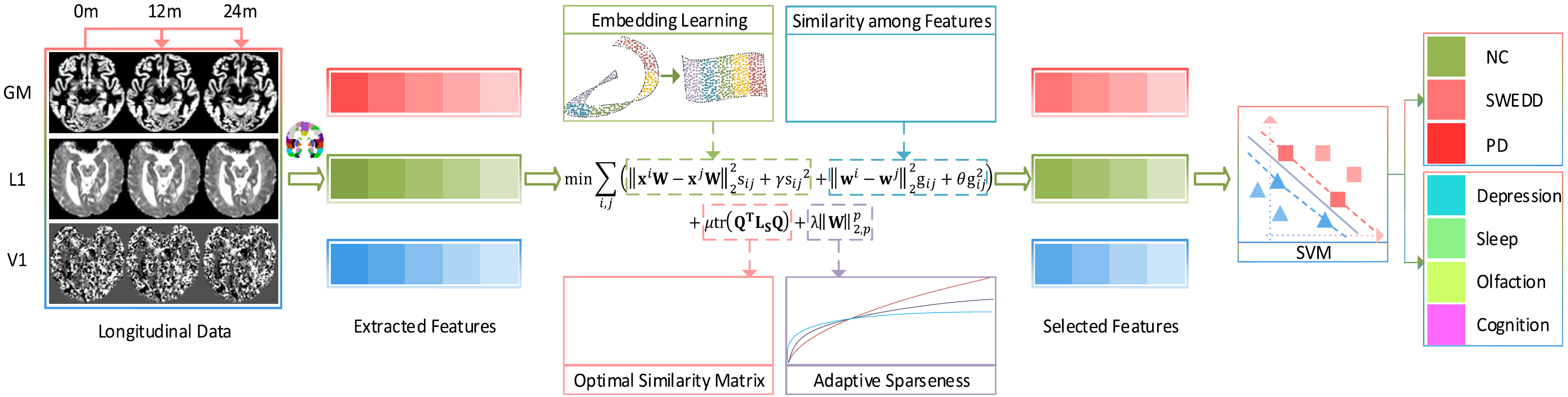

Fig. 1. Illustration of the proposed approach through united embedding and sparse regression.
Abstract
Parkinson's disease (PD) is known as an irreversible neurodegenerative disease that mainly affects the patient's motor system. Early classification and regression of PD are essential to slow down this degenerative process from its onset. In this paper, a novel adaptive unsupervised feature selection approach is proposed by exploiting manifold learning from longitudinal multimodal data. Classification and clinical score prediction are performed jointly to facilitate early PD diagnosis. Specifically, the proposed approach performs united embedding and sparse regression, which can determine the similarity matrices and discriminative features adaptively. Meanwhile, we constrain the similarity matrix among subjects and exploit the L2,p norm to conduct sparse adaptive control for obtaining the intrinsic information of the multimodal data structure. An effective iterative optimization algorithm is proposed to solve this problem. We perform abundant experiments on the Parkinson's Progression Markers Initiative (PPMI) dataset to verify the validity of the proposed approach. The results show our approach boosts performance on the classification and clinical score regression of longitudinal data and surpasses the state-of-the-art approaches.
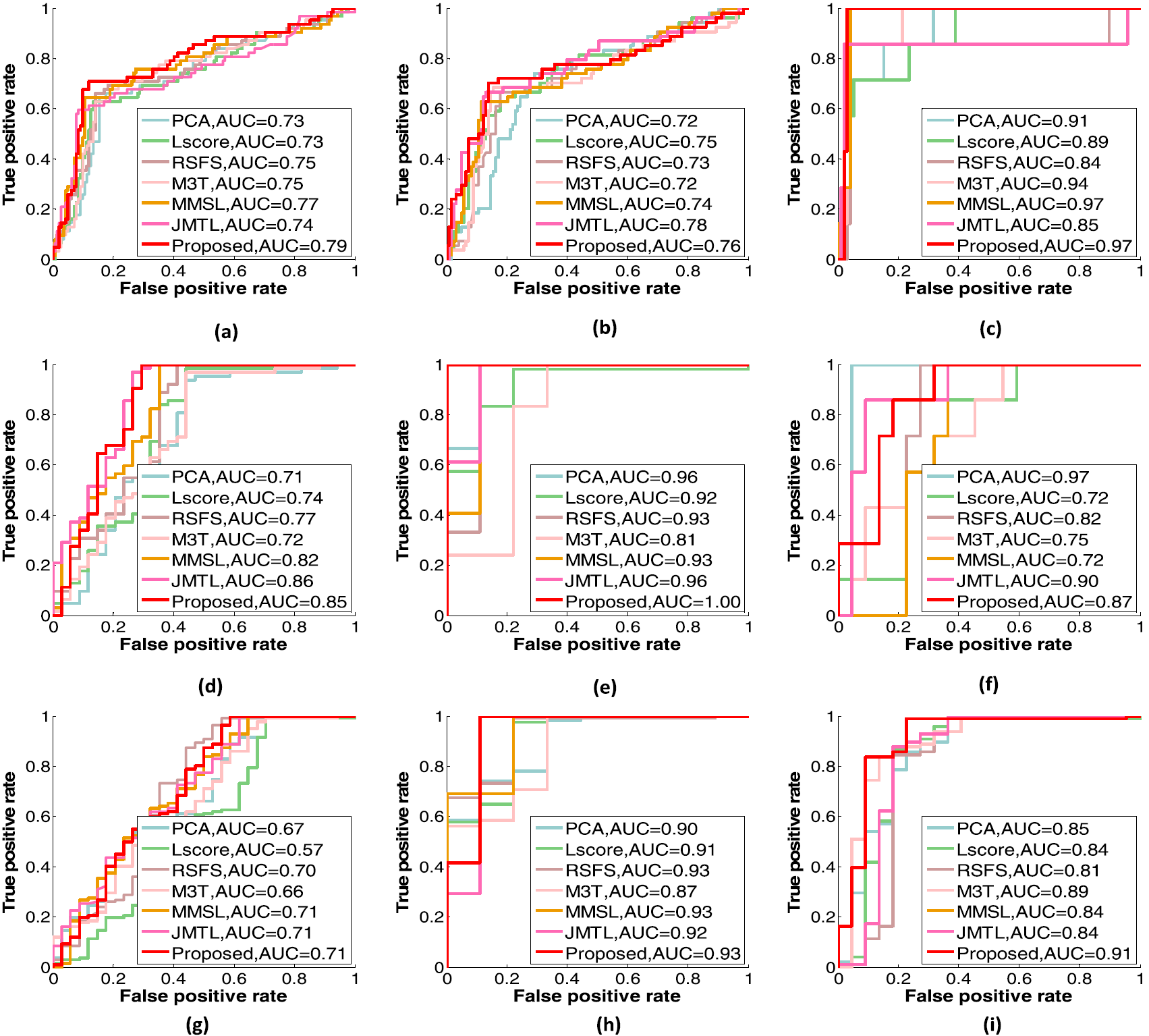
Fig. 2. Comparison of ROC curves for the competing approaches on longitudinal multimodal data.
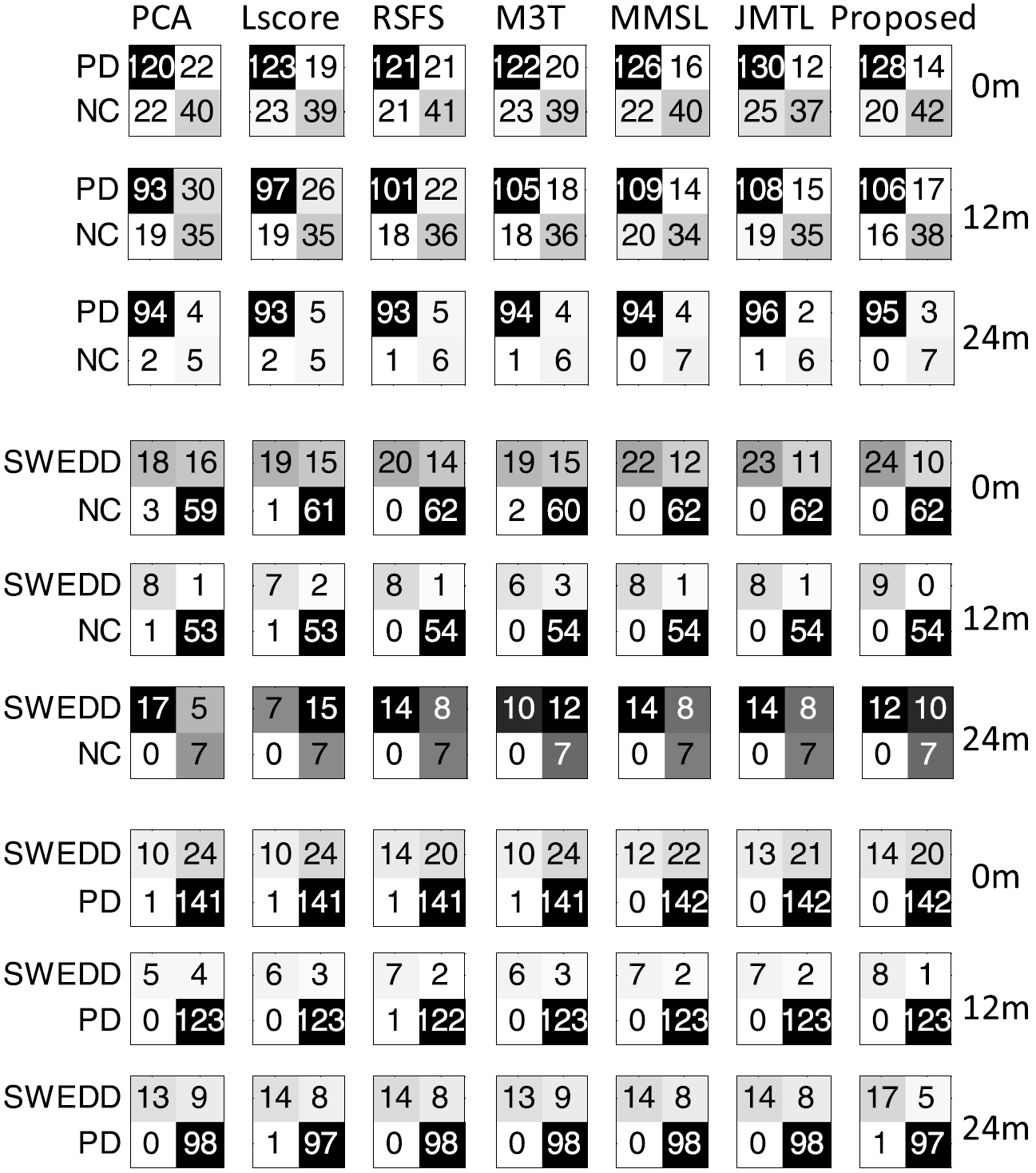
Fig. 3. Confusion matrices for the competing approaches on longitudinal data.
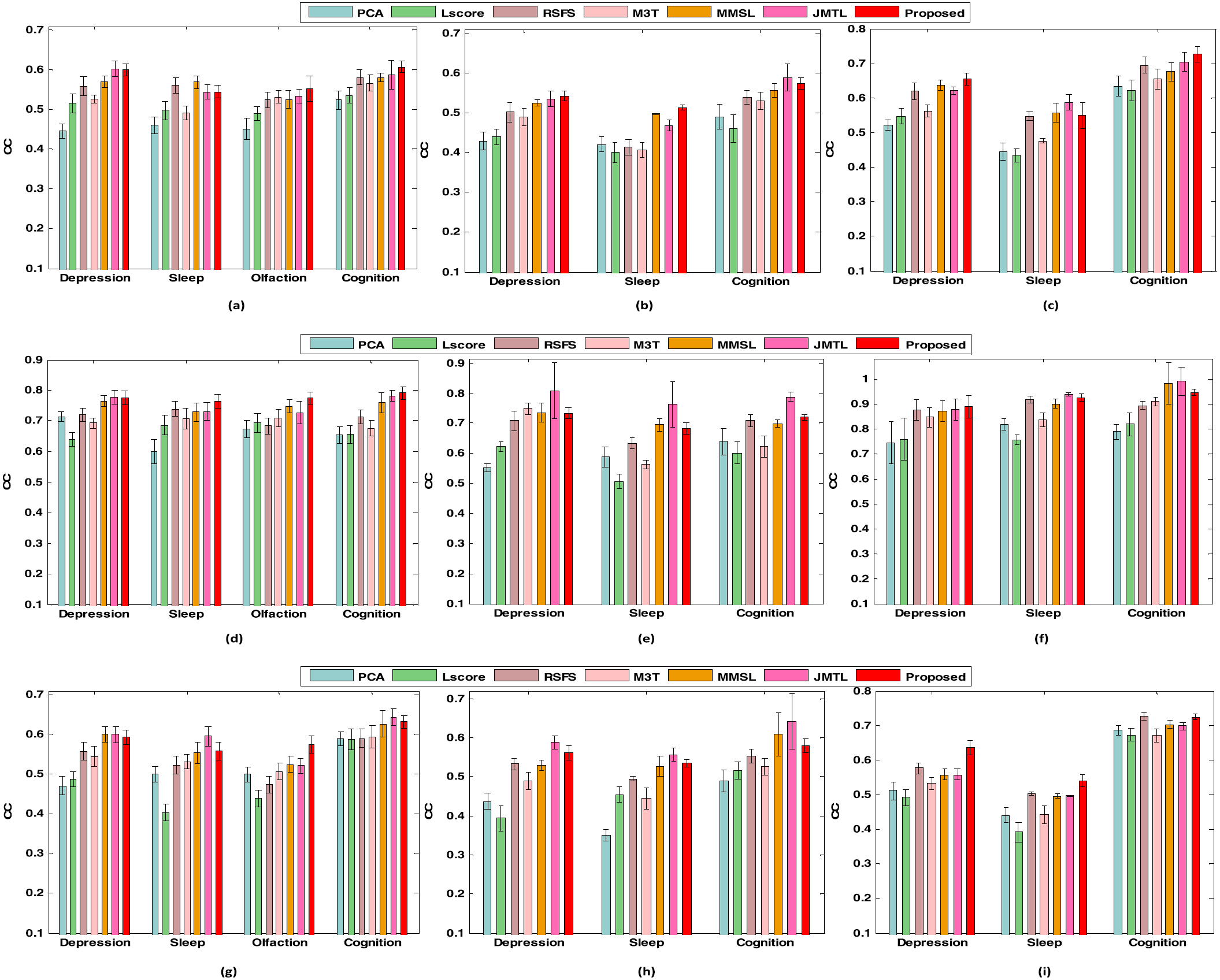
Fig. 4. Regression performance for the competing approaches on longitudinal multimodal data.
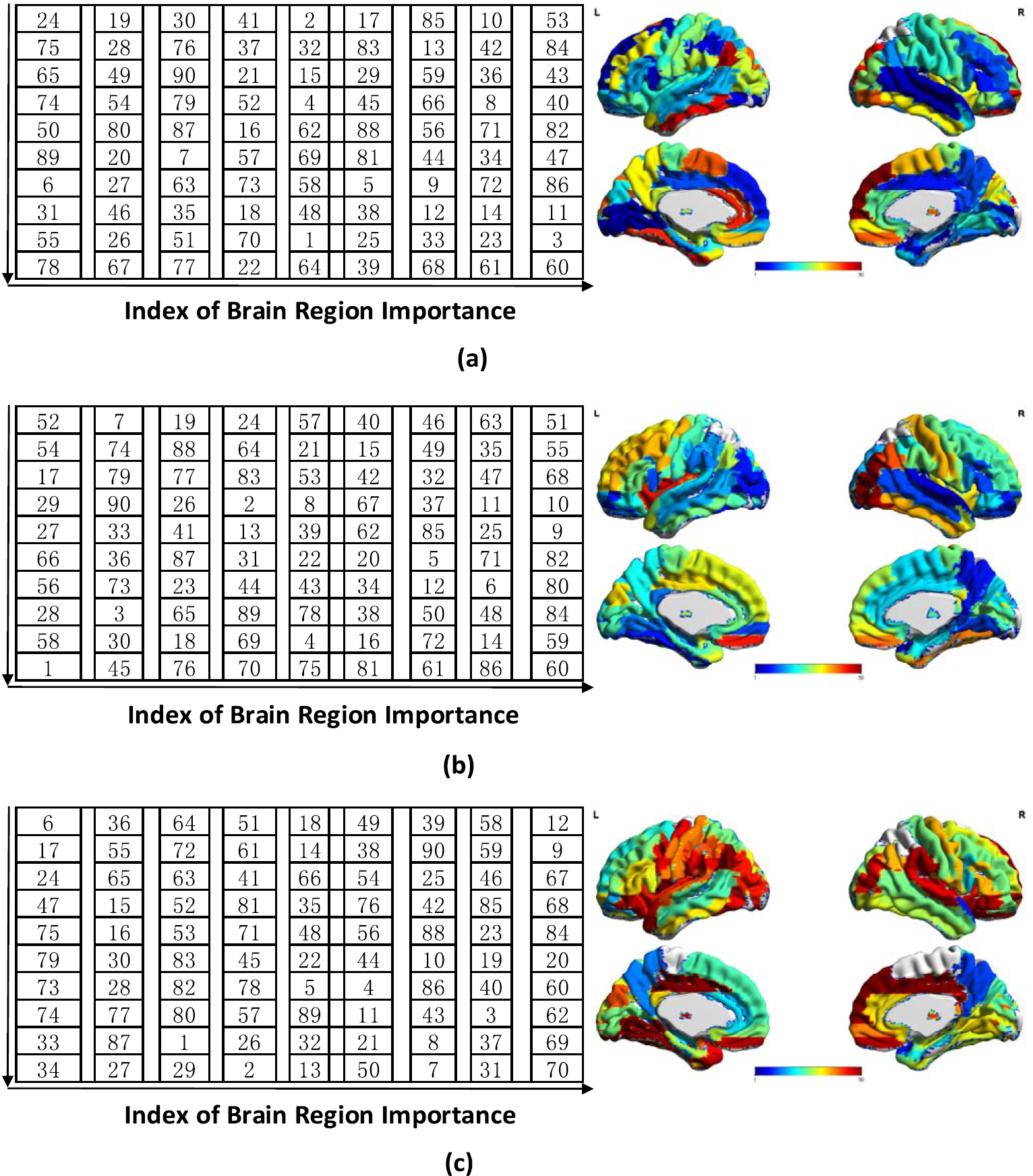
Fig. 5. Index of brain region importance and corresponding visual representation on the baseline data (Note that the importance of brain regions is sorted from top to bottom and left to right in the left table).
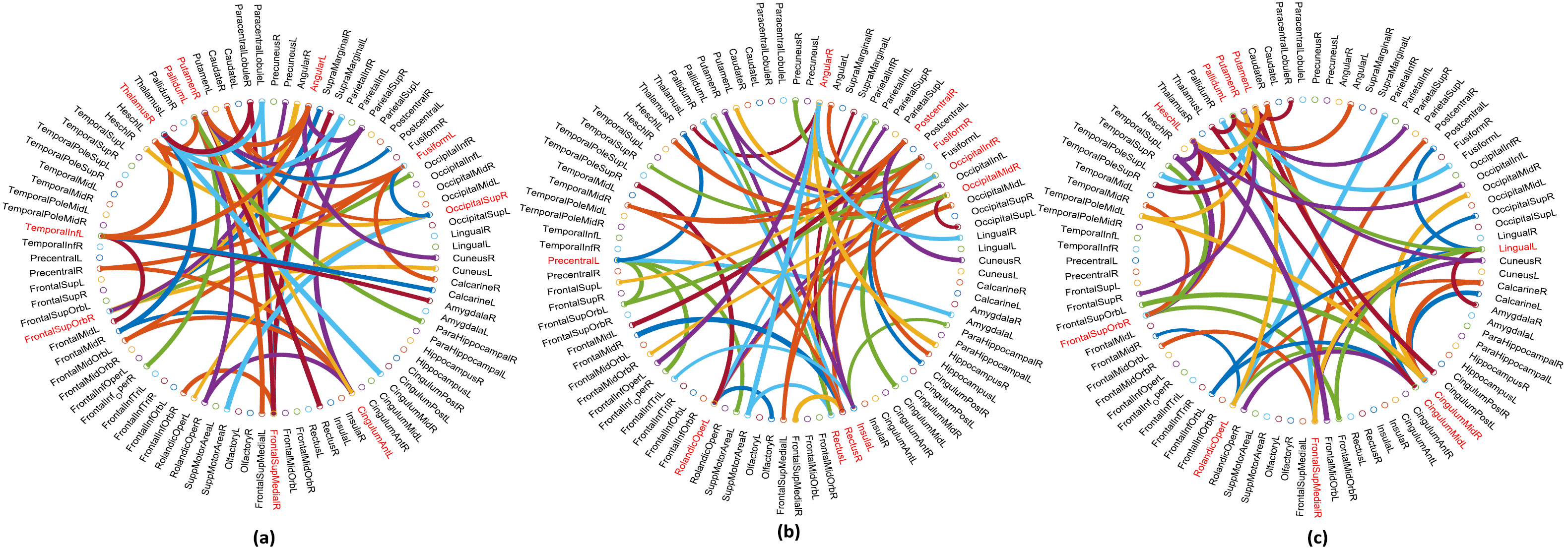
Fig. 6. Correlation maps between top ten brain regions with red fonts and other brain regions with black fonts on the baseline data.

Fig. 7. Top twenty brain regions connectivity on longitudinal data.
Acknowledgements
This work was supported partly by National Natural Science Foundation of China (Nos. 61871274, 61772118, 61801305, 81571758, and 61950410615), National Natural Science Foundation of Guangdong Province (Nos. 2020A1515010649 and 2019A1515111205), (Key) Project of Department of Education of Guangdong Province (No. 2019KZDZX1015), Guangdong Laboratory of Artificial-Intelligence and Cyber-Economics (SZ), Shenzhen Peacock Plan (Nos. KQTD2016053112051497 and KQTD2015033016104926), Shenzhen Key Basic Research Project (Nos. JCYJ20190808165209410, 20190808145011259, JCYJ20180507184647636, GJHZ20190822095414576, JCYJ20170302153337765, JCYJ20170302150411789, JCYJ201703 02142515949, GCZX2017040715180580, GJHZ20180418190529516, and JSGG20180507183215520), Hong Kong Research Grants Council (No. PolyU 152035/17E), AFF is supported by the RAEng Chair in Emerging Technologies (CiET1819/19).
Bibtex
@article{0Parkinson,
title={Parkinson's Disease Classification and Clinical Score Regression via United Embedding and Sparse Learning From Longitudinal Data}, author={ Huang, Z. and Lei, H. and Chen, G. and Frangi, A. F. and Lei, B. },
journal={IEEE transactions on neural networks and learning systems},
volume={PP},
}
Downloads