International Conference on Research and Development in Information Retrieval (SIGIR)
Mingyang Chen1 Junda Lu1 Yi Wang2 Jianbin Qin3 Wei Wang1
1University of New South Wales 2Dongguan University of Technology 3Shenzhen Institute of Computing Sciences
ABSTRACT
There is an increasing interest in studying adversarial attacks on image retrieval systems. However, most of the existing attack methods are based on the white-box setting, where the attackers have access to all the model and database details, which is a strong assumption for practical attacks. The generic transfer-based attack also requires substantial resources yet the effect was shown to be unreliable.In this paper, we make the first attempt in proposing a query efficient decision-based attack framework for the image retrieval(DAIR) to completely subvert the top-retrieval results with humanimperceptible perturbations. We propose an optimization-based method with a smoothed utility function to overcome the challenging discrete nature of the problem. To further improve the query efficiency, we propose a novel sampling method that can achieve the transferability between the surrogate and the target model efficiently. Our comprehensive experimental evaluation onthe benchmark datasets shows that our DAIR method outperformssignificantly the state-of-the-art decision-based methods. We alsodemonstrate that real image retrieval engines (Bing Visual Search and Face++ engines) can be attacked successfully with only several hundreds of queries.
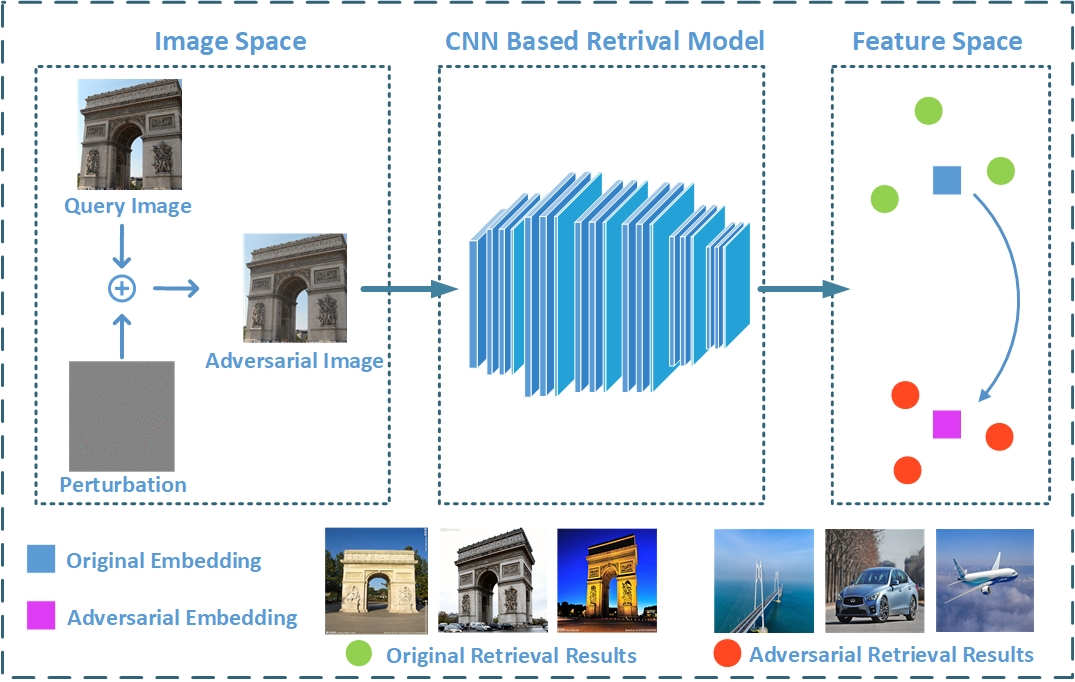
Figure 1: Overview of attacking image retrieval systems.
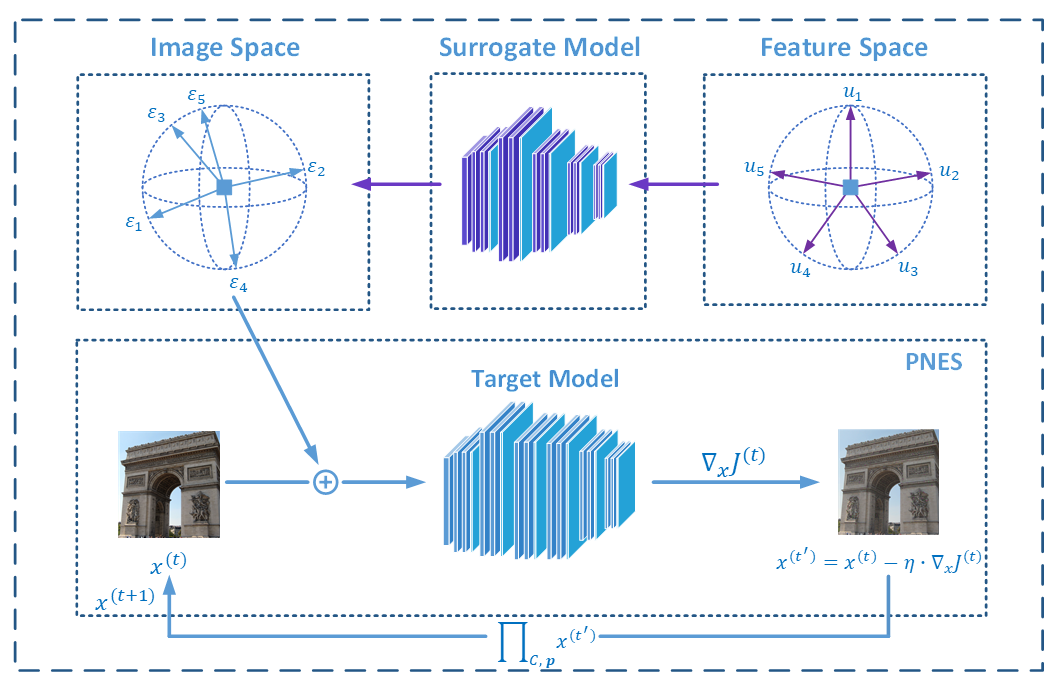
Figure 2: Overview of combining the self-adaptive sampling method with the PNES framework.
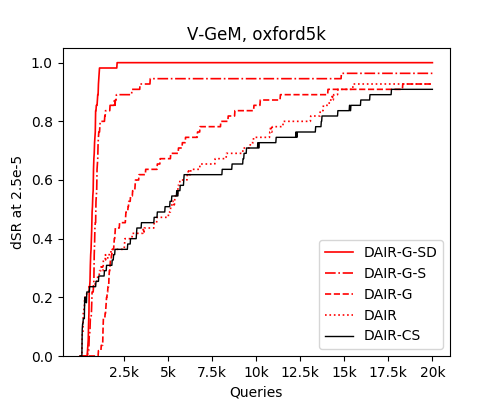
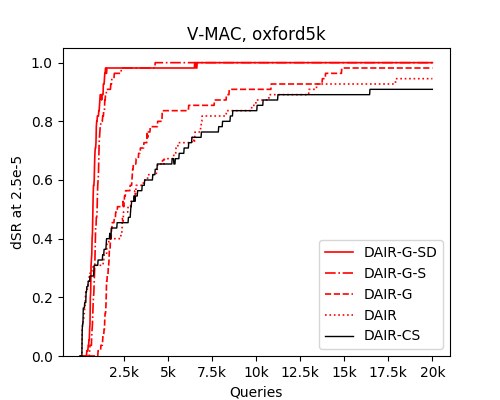
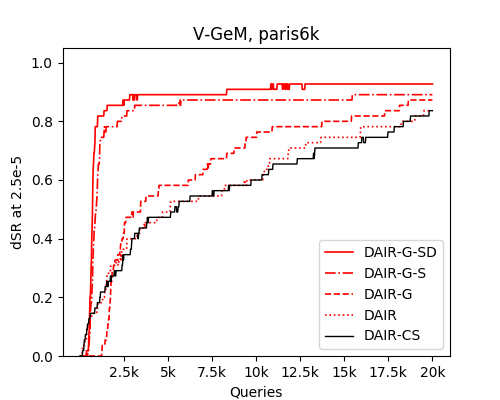
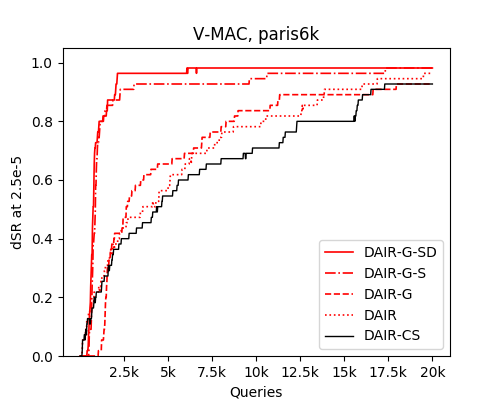
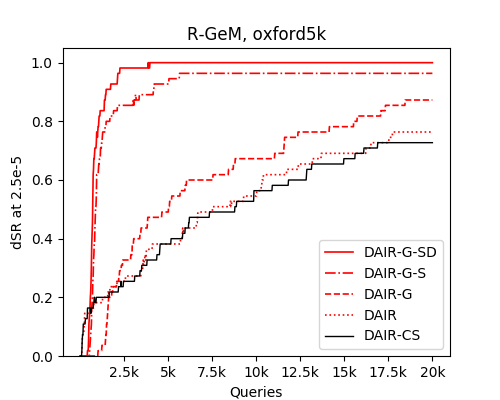
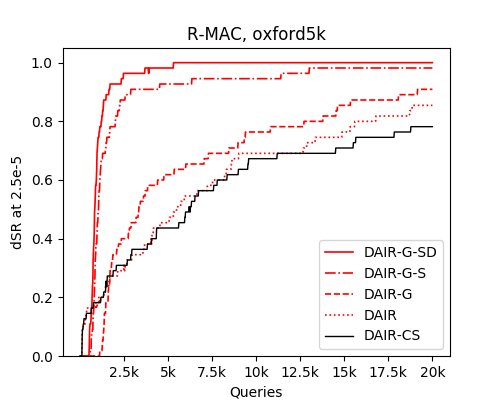
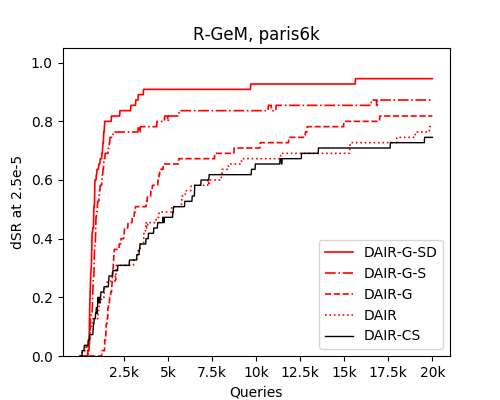
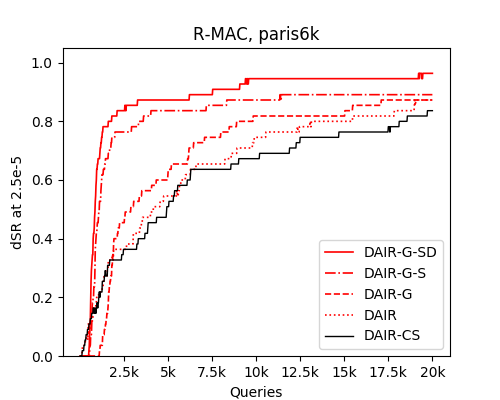
Figure 3: Plots of distortion-based success rates versus the number of queries under the distortion threshold of 2.5 × 10−5

Figure 4: An example of attacking the Bing Visual Search API. Note that images in red frames indicate that they are different type of product (i.e., carpet) to the original query image (i.e., wardrobe).
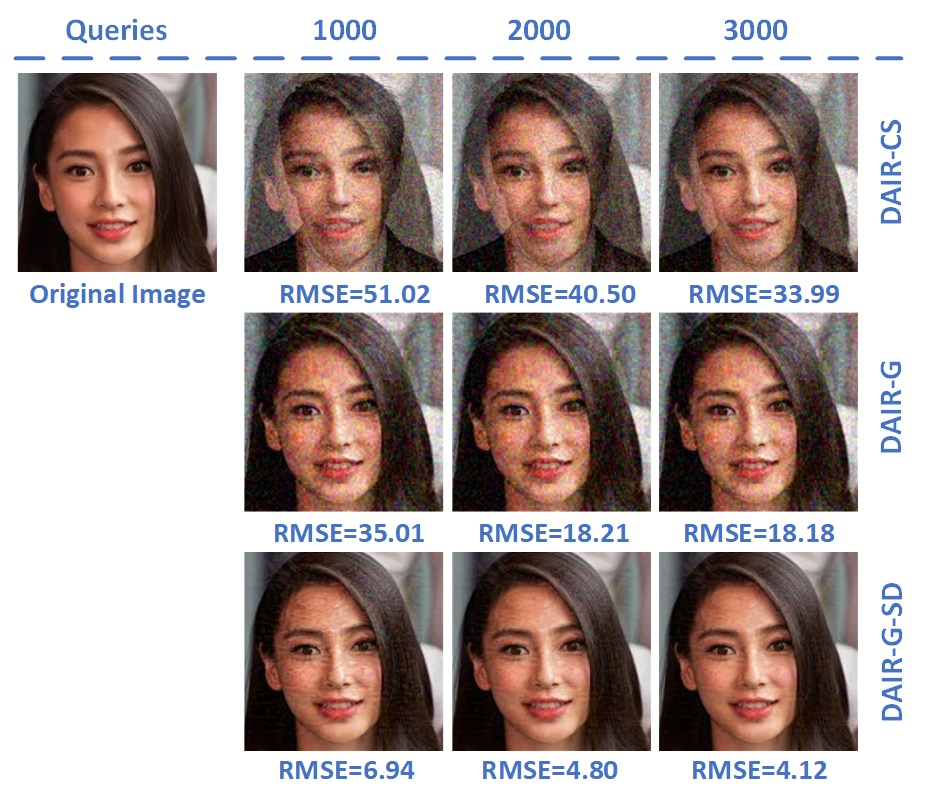
Figure 5: An example of attacking the Face++ Face Search API under different number of queries
Acknowledgements
The work was supported in part by ARC Discovery Projects 180103411 and 220101762, Natural Science Foun-dation of China (grant no.61876038), Dongguan Social Science and Technology Development Key Project (grant no. 2020507140146), Dongguan University of Technology under project (grant no. KCYKYQD2017003), Guangdong Basic and Applied Basic Research Foundation (grant no. 2020B1515120028), and Guangdong Peral River Recruitment Program of Talents (grant no. 2019ZT08X603).
BibTeX
@inproceedings{
DBLP:conf/sigir/ChenLWQW21,
author = {Mingyang Chen and Junda Lu and Yi Wang and Jianbin Qin and Wei Wang},
editor = {Fernando Diaz and Chirag Shah and Torsten Suel and Pablo Castells and Rosie Jones and Tetsuya Sakai},
title = {{DAIR:} {A} Query-Efficient Decision-based Attack on Image Retrieval Systems},
booktitle = {{SIGIR} '21: The 44th International {ACM} {SIGIR} Conference on Research and Development in Information Retrieval, Virtual Event, Canada, July 11-15, 2021},
pages = {1064--1073},
publisher = {{ACM}},
year = {2021},
url = {https://doi.org/10.1145/3404835.3462887},
doi = {10.1145/3404835.3462887},
timestamp = {Fri, 04 Feb 2022 10:59:22 +0100}, biburl = {https://dblp.org/rec/conf/sigir/ChenLWQW21.bib},
bibsource = {dblp computer science bibliography, https://dblp.org}
}
Downloads