IEEE Transactions on Parallel and Distributed Systems (TPDS)
Amelie Chi Zhou1 Bingkun Shen1 Yao Xiao1 Shadi Ibrahim2 Bingsheng He3
1Shenzhen University 2Inria 3National University of Singapore
Abstract
Graph processing is an emerging computation model for a wide range of applications and graph partitioning is important for optimizing the cost and performance of graph processing jobs. Recently, many graph applications store their data on geo-distributed datacenters (DCs) to provide services worldwide with low latency. This raises new challenges to existing graph partitioning methods, due to the multi-level heterogeneities in network bandwidth and communication prices in geo-distributed DCs. In this article, we propose an efficient graph partitioning method named Geo-Cut, which takes both the cost and performance objectives into consideration for large graph processing in geo-distributed DCs. Geo-Cut adopts two optimization stages. First, we propose a cost-aware streaming heuristic and utilize the one-pass streaming graph partitioning method to quickly assign edges to different DCs while minimizing inter-DC data communication cost. Second, we propose two partition refinement heuristics which identify the performance bottlenecks of geo-distributed graph processing and refine the partitioning result obtained in the first stage to reduce the inter-DC data transfer time while satisfying the budget constraint. Geo-Cut can be also applied to partition dynamic graphs thanks to its lightweight runtime overhead. We evaluate the effectiveness and efficiency of Geo-Cut using real-world graphs with both real geo-distributed DCs and simulations. Evaluation results show that Geo-Cut can reduce the inter-DC data transfer time by up to 79 percent (42 percent as the median) and reduce the monetary cost by up to 75 percent (26 percent as the median) compared to state-of-the-art graph partitioning methods with a low overhead.
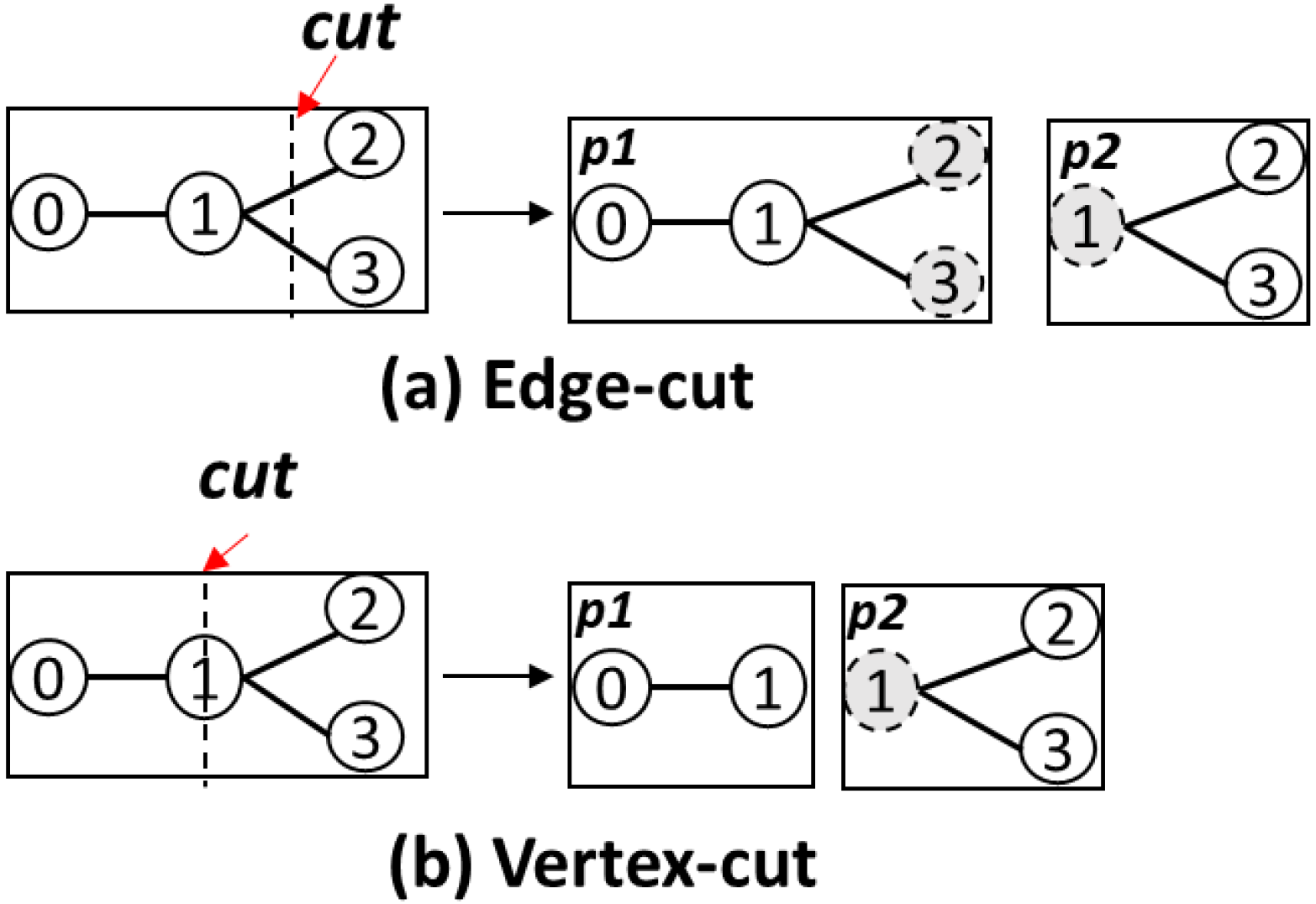
Fig. 1: Illustrations of (a) edge-cut and (b) vertex-cut. Shaded vertices represent ghosts and mirrors respectively.
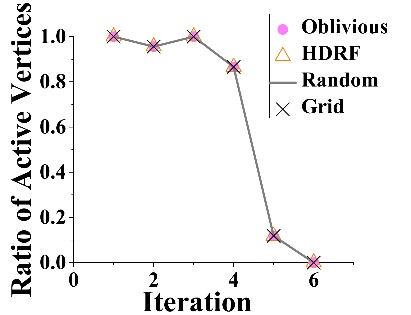
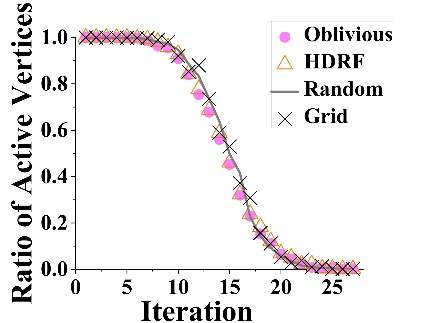
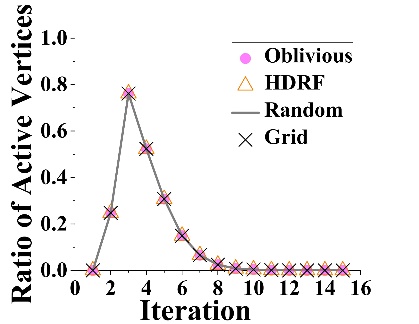
Fig. 3: Convergence analysis of PageRank, SSSP and SI under different strategies. The convergence speeds under the four partitioning strategies are exactly the same for SSSP and SI.
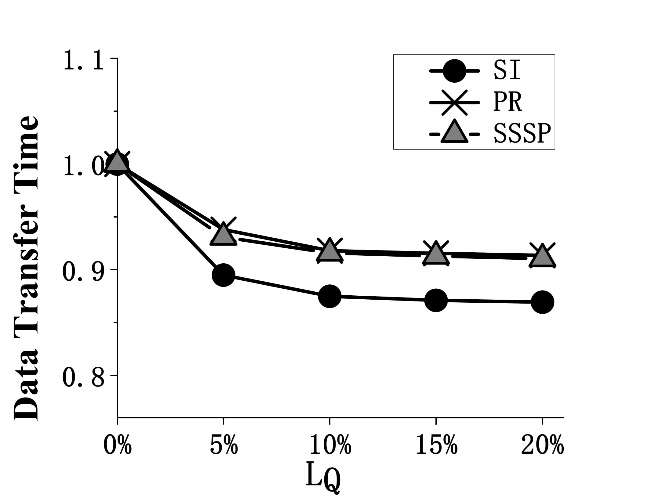
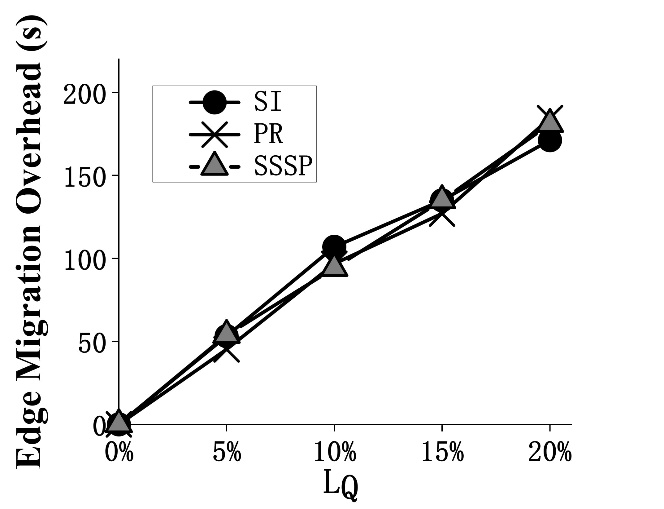
Fig. 14: Sensitivity study of LQ on Amazon EC2 using LJ.
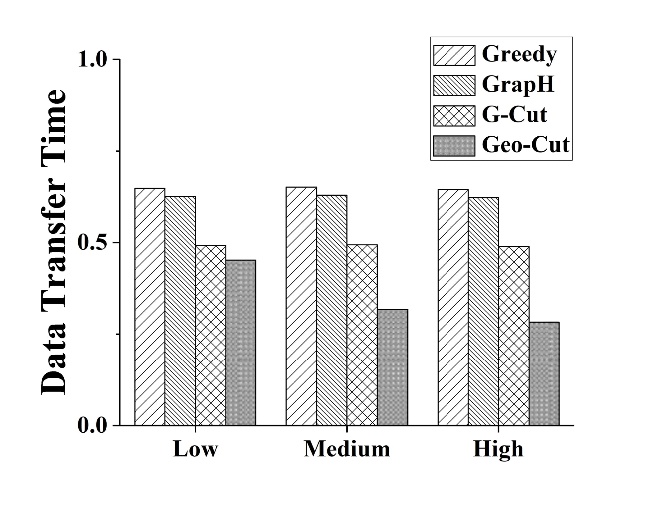
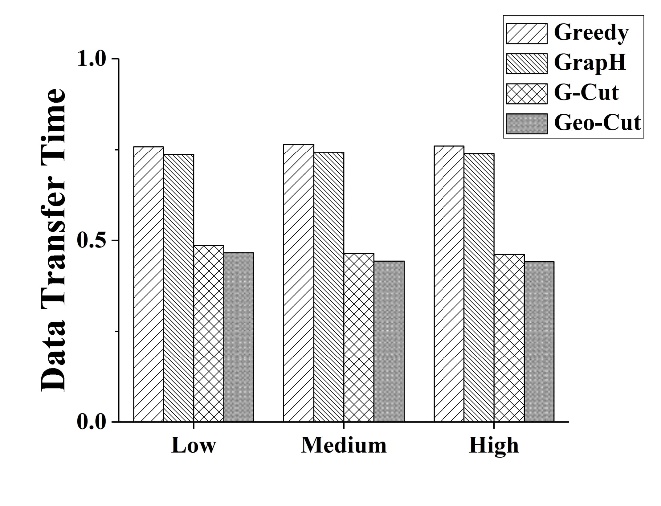
Fig. 15: Normalized inter-DC data transfer time under different bandwidth heterogeneities using Livejournal.
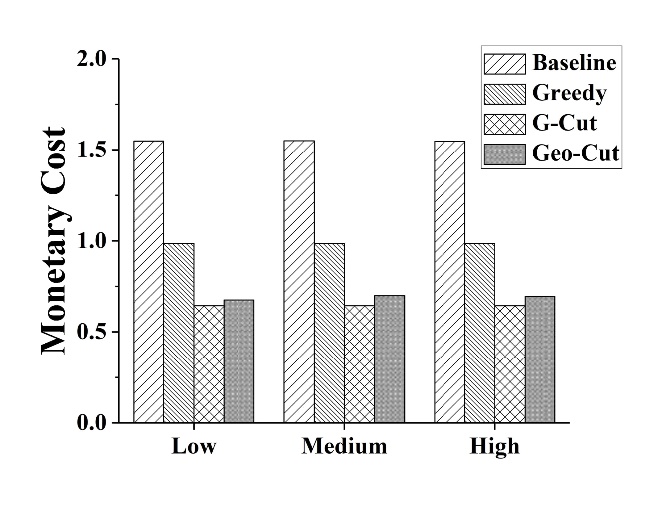
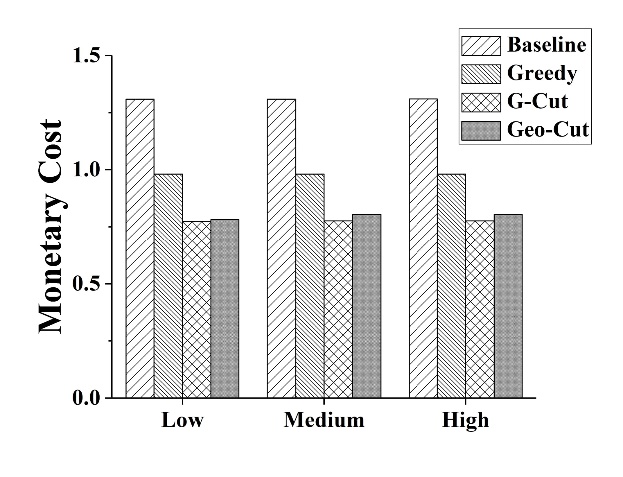
Fig. 16: Normalized inter-DC data transfer cost under different bandwidth heterogeneities using Livejournal.
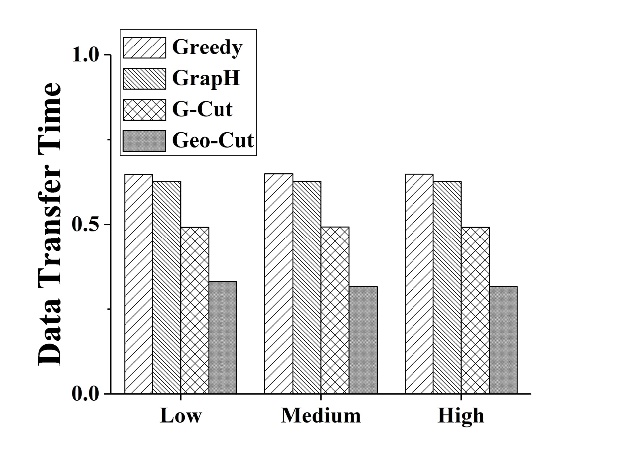
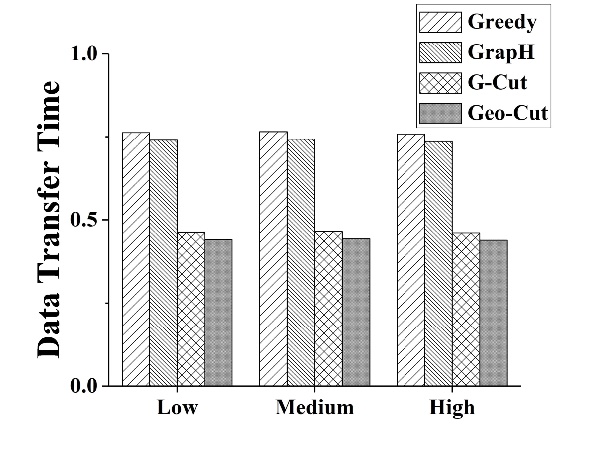
Fig. 17: Normalized inter-DC data transfer time under different price heterogeneities using Livejournal.
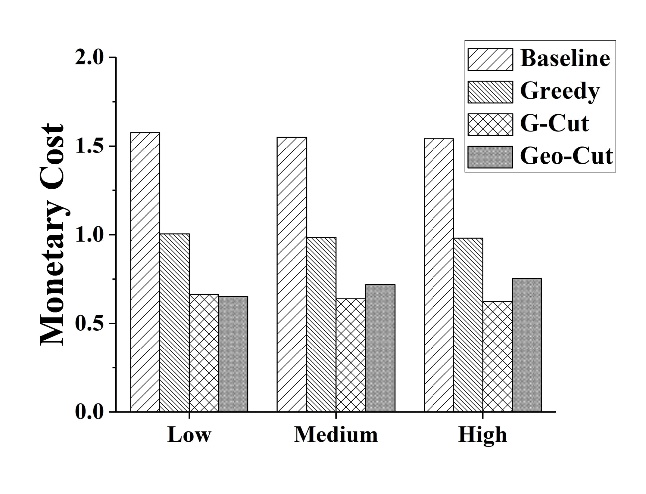
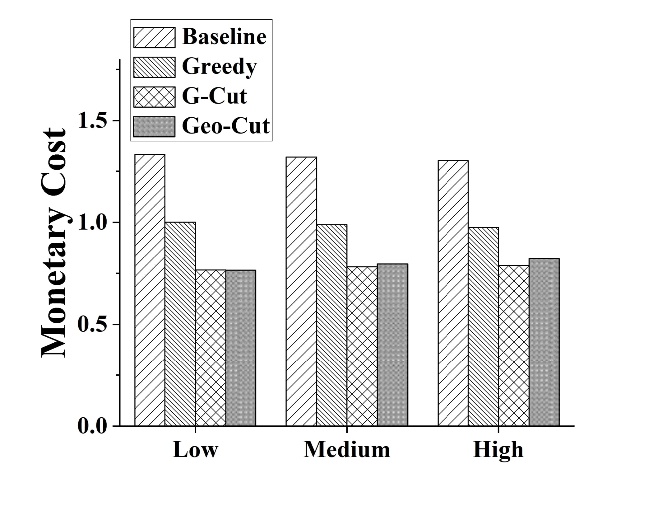
Fig. 18: Normalized inter-DC data transfer cost under different price heterogeneities using Livejournal.
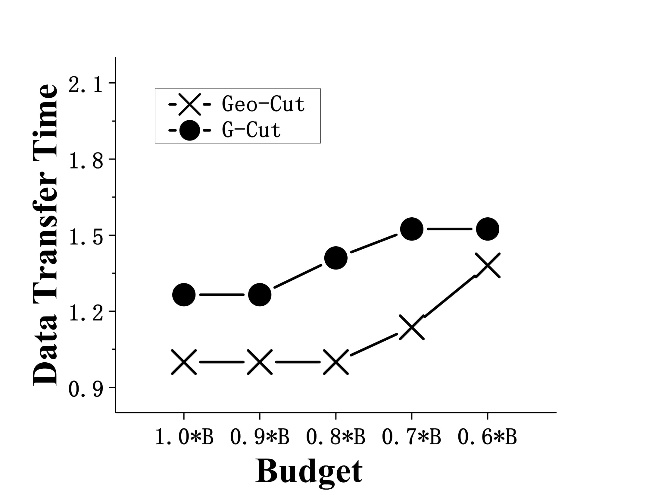
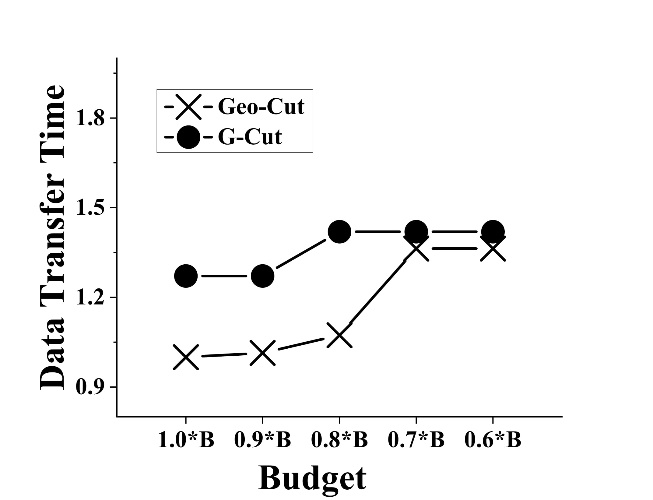
Fig. 19: Normalized inter-DC data transfer time under different budget constraints.
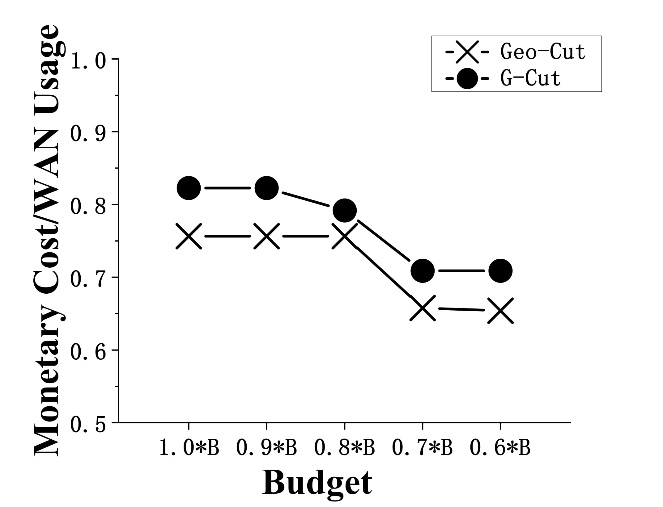
Fig. 20: Monetary cost/WAN usage under different budget constraints.
Acknowledgements
This work was supported in part by the National Natural Science Foundation of China (No. 61802260), the Guangdo
ng Natural Science Foundation Grant Nos. 2018A030310440 and 2019A1515012053, the Shenzhen Science and Technology Foundation Grant No. JCYJ20180305125737520, the Natural Science Foundation of SZU Grant Nos. 827-000370, 827-000175 and 860-000002110319, the Guangdong Province Key Laboratory of Popular High Performance Computers Grant No.2017B030314073, the ANR KerStream project (ANR-16-CE25-0014-01), and the Stack/Apollo connect talent project. Bingsheng He’s research is partly supported by aMoE AcRF Tier 1 Grant (T1 251RES1610) in Singapore and National Natural Science Foundation of China Grant No. 61929103.
Bibtex
@ARTICLE{8911231,
author={Zhou, Amelie Chi and Shen, Bingkun and Xiao, Yao and Ibrahim, Shadi and He, Bingsheng},
journal={IEEE Transactions on Parallel and Distributed Systems},
title={Cost-Aware Partitioning for Efficient Large Graph Processing in Geo-Distributed Datacenters},
year={2020},
volume={31},
number={7},
pages={1707-1723},
}
Downloads